Manufacturing Excellence Utilizing a Life Cycle Approach

The emergence of “big data” has allowed pharmaceutical organizations to harness the vast amount of information they generate. By collecting equipment, facility, and manufacturing data (process parameters, calibration, qualification, environmental conditions, etc.) companies can improve process development, apply continuous improvement initiatives, and reduce failures on the floor.
Knowledge gained on sufficiently similar products and processes at commercial scale can further provide confidence of product quality. Process validation guidance from the US Food and Drug Administration (FDA), 1 along with process validation guidelines 2 and Annex 15 3 from the European Medicines Agency (EMA) mandate commercialization only when data has established a high degree of product and process understanding and has demonstrated adequate process controls. FDA’s Q8, Q9, and Q10 Questions and Answers (R4) 4 further clarify that like the product itself, process validation also has life cycle stages:
- Stage 1: Process design
- Stage 2: Process qualification
- Stage 3: Continued process verification (CPV)
Knowledge management (KM) is a method of capturing, storing, distributing, and utilizing explicit, implicit, and tacit knowledge to improve product knowledge and process understanding.6 KM is also an enabler for implementing ICH Q10, “Pharmaceutical Quality System,” 5 and supports manufacturing excellence by assuring product realization, robust control strategies, and continuous improvement.
Finally, KM applications are imperative to support a life cycle approach for any given product or process. This article reviews three case studies in which effective product and process KM from all three stages of the process validation life cycle enabled risk-based and data-driven decision-making.
Case Studies
1. Addressing variability in uniformity for a legacy product
During annual product quality review of an immediate-release solid-dose product, the Quality Unit observed out-of-statistical-control (OOSC)7 results during powder blend uniformity (BU) trending. Berman, et al. suggest that if the product passes but the blend fails, further evaluation is necessary as a significant sample error may be affecting the results. 8 We initiated an investigation to determine the likelihood of blend segregation, including review of the extended content uniformity testing results. The data and tight relative standard deviation indicated that powder blend segregation was not the most likely root cause; sampling bias was suspected. Per Berman, the results are applicable to statistical inference B, which is:
… indicative of sampling bias. Significant differences exist between the means of the blender and product indicating a high probability the samples were not taken from the same population. The standard deviation of the blender is greater than that of the product providing further evidence of sampling bias. These are the conditions most frequently seen when sampling bias is occurring. 8
It was essential, therefore, to confirm that the sample size provided adequate representation of the entire blend batch.
Purutyan and Carson suggest that sample size has a profound impact on the variable blend uniformity results.9 Since the product was a legacy molecule with minimal stage 1 quality by design data on blend samplingsizes, understanding application of sample sizes for similar products was critical. Because data was available across multiple products at all stages of the life cycle, the KM system was able to perform an ad hoc query.
Sample size, blend data, and associated information came from sources such as the laboratory information management system (LIMS) (i.e., results), quality management system (QMS) (i.e., investigations), and enterprises resource planning system (ERP) (i.e., batch genealogy). All were integrated into the life cycle KM system. Sample sizes used for similar formulations with similar physical characteristics of the blend were identified and used.
A verification batch performed with the suggested sample size was successful, which confirmed the investigation hypothesis of sampling bias. A new sample size was suggested for future batches. In addition, parameters that affect BU results, such as particle-size distribution, were evaluated to determine if there was a correlation. Application of existing data eliminated the need for experiments, prevented delays, reduced failure, and limited additional cost of material. The approach also reduced the need for production resources and ensured continued commercial supply of the product.
2. Site transfer and scale-up
For a site transfer of an existing solid-dose formulation, the manufacturing process and equipment at the sending site were compared to those at the receiving site. Review of the formulation, equipment comparability, and risk assessment of the proposed process showed that the blend process should be scaled-up to meet volume demands, because multiple similar scale bin blenders were not available. It was further determined that apart from scale, the blend process, bin blender geometry, and mixing dynamics between the proposed bin and the bin blender at the sending site were similar. As a result, scale-up risk therefore was estimated to be low.
We therefore decided to leverage the life cycle KM system to understand the process parameters of similar products to establish optimal blend process parameters prior to a demonstration batch. Identifying products and processes with comparable formulation (percent active), raw material physical characteristics, blend batch size, bin fill, blend bulk density, and blend uniformity results allowed review of potential issues. The blend process parameters were established and confirmed during the demonstration batch.
The ability to retrieve essential information through the life cycle KM system negated the need for additional manufacturing floor trials and additional resources. Data compilation, analysis, and trending were performed by the life cycle KM system, integrating LIMS, QMS, and ERP. This ensured that blend parameters were based on sound science and overall product and process understanding prior to initiating stage 1 verification studies. Per FDA process validation guidance, it is not typically necessary to explore the entire operating range at a commercial scale if assurance can be provided from credible experience with sufficiently similar products and processes. 2
- 1US Food and Drug Administration. Guidance for Industry. “Process Validation: General Principles and Practices” January 2011. http://www.fda.gov/downloads/Drugs/Guidance s/UCM070336.pdf
- 2 a b European Medicines Agency. “Guideline on Process Validation for Finished Products—Information and Data to Be Provided in Regulatory Submissions.” 21 November 2016. http://www.ema.europa.eu/docs/en_GB/document_library/Scientific_guideline/2014/02/WC500162136.pdf
- 3European Commission. Directorate General for Health and Food Safety. EudraLex, Volume 4, Annex 15: “Qualification and Validation.” 30 March 2015. http://ec.europa.eu/health/files/eudralex/vol-4/2015-10_annex15.pdf
- 4US Food and Drug Administration. Guidance for Industry. “Q8, Q9, and Q10 Questions and Answers (R4).” November 2011, ICH Revision 1. http://www.fda.gov/downloads/Drugs/.../Guidances/UCM210822.pdf
- 6Davenport, T., and L. Prusak. Working Knowledge: How Organizations Manage What They Know. Boston, MA: Harvard Business School Press, 1998.
- 5International Conference on Harmonization of Technical Requirements for Registration of Pharmaceuticals for Human Use. “Pharmaceutical Quality System: Q10.” 4 June 2008. http://www.ich.org/fileadmin/Public_Web_Site/ICH_Products/Guidelines/Quality/Q10/Step4/Q10_Guideline.pdf.
- 7Flexman G., et al. Parenteral Drug Association. Technical Report #59: “Utilization of Statistical Methods for Production Monitoring.” Bethesda, MD: PDA, 2012.
- 8 a b Berman, J., et al. Technical Report # 25: “Blend Uniformity Analysis: Validation and In-Process Testing”. Bethesda, MD: PDA, 1997.
- 9Purutyan H., and J. W. Carson. “Predicting, Diagnosing, and Solving Mixture Segregation Problems.” Powder and Bulk Engineering (January 2007). http://www.powderbulk.com/enews/2013/whitepaper/jenike.pdf
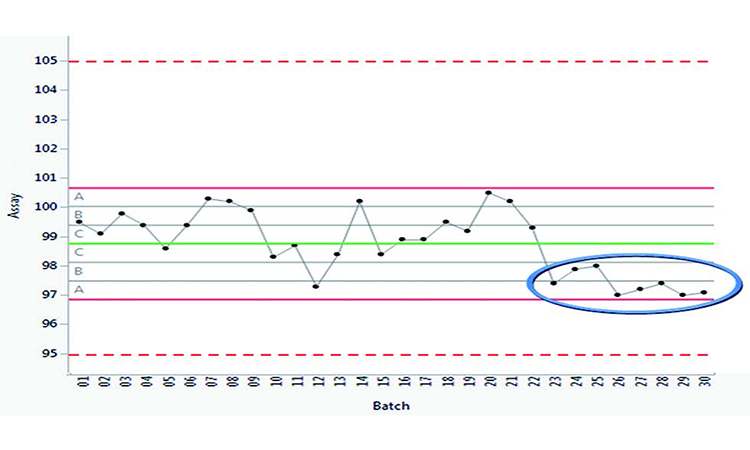
3. CPV trend
Figure 1 shows continued monitoring of assay results, a critical quality attribute (CQA) for an immediate-release solid-dose product, where the specification is 95.0%–105.0%. The control chart includes zoning by a standard deviation of 1 sigma. This identifies unnatural patterns by applying commonly accepted test rules.* 10
Figure 1 depicts eight consecutive assay data points on one side of the mean value (WECO rule 4). This highlights an unnatural pattern, indicating small sustained shifts or trends; the results, however, are well within the specification limits. Assay results were retrieved from LIMS and trended in the life cycle KM system. Further investigation identified a root cause: Corresponding analysis of weight variation indicated that batches were being compressed below target weight (although still within specification). Corrective and preventive measures were enacted to ensure target weight was maintained prior to further processing, resulting in an uninterrupted production cycle.
Trending near and/or real-time quality attribute and process parameter data to ensure compliance with FDA process validation guidance 2 is of paramount value, adding assurance that the process remains in a state of control during routine commercial production of drug products. Through life cycle stage 1, critical material attributes and CQAs are established; critical process parameters (CPPs) and process control strategies are defined. CPPs and CQAs are verified in stage 2. As the product moves to stage 3, the body of data grows significantly. 11 In stages 3A and 3B, CPV data continues to be generated until the product is discontinued. 17 Control mechanisms such as ensuring notifications for out-of-trend or OOSC data are established as part of the CPV program. Trend detection is easily visible and alerts can be generated for further evaluation. Special attention is required to detect false alerts—those not process related—to curb overreaction and focus only on significant trends. As data is available in near real time, it allows immediate action upon signal detection.
Information in the life cycle KM system may include elements such as detailed manufacturing processing stages, equipment used, and process parameters, which can generate automated outputs such as manufacturing process flow charts. A well-maintained product history, including change control summaries, validation statuses, investigations, complaints, field alerts, and stability data, lends itself well to an automated annual product quality review report. 12 Integrating typical pharmaceutical document, content, and workflow management systems such as LIMS, ERP, and QMS are essential to managing the process validation life cycle stages. 13 Data must be accessible, gathered, interfaced, and delivered to continually support all life cycle stages. New FDA guidance on emerging technology applications 14 promotes the adoption of innovative technology; other regulatory agencies have provided further clarity on data integrity, cGMP compliance, 15 and current thinking on data creation and handling.
* Western Electric (WECO) rules, Nelson tests, ISO 2859 tests, Boeing ASQ rules, and Trietsch rules
- 10Noskievicova D. “Complex Control Chart Interpretation.” International Journal of Engineering Business Management 5, no. 13 (March 2013). http://hrcak.srce.hr/file/237260
- 11Mylopoulos, J., et al. “Building Knowledge Base Management Systems.” VLDB Journal (1996). http://www.cs.toronto.edu/~jm/Pub/VLDBJ96.pdf
- 17Sayeed-Desta, N., et al. “Assessment Methodology for Process Validation Lifecycle Stage 3A.” AAPS PharmSciTech 28, no. 5 (July 2017): 1881 -1886.
- 12Pazhayattil, A. “Annual Product Reviews: How to Conduct an Effective Annual Product Quality Review.” 1 February 2012. http://www.pharmamanufacturing.com/articles/2012/018/
- 13Wipro, CMS Watch, Smigiel Consulting Group, and Hartman Communicatie. ECM3: Maturity Model Version 1.0. 18 February 2009. https://ecmmaturity.files.wordpress.com/2009/02/ec3m-v01_0.pdf
- 14US Food and Drug Administration. Draft Guidance. “Advancement of Emerging Technology Applications to Modernize the Pharmaceutical Manufacturing Base.” December 2015. http://www.fda.gov/downloads/Drugs/GuidanceComplianceRegulatoryInformation/Guidances/UCM478821.pdf
- 15US Food and Drug Administration. Draft Guidance. “Data Integrity and Compliance with cGMP.” April 2016. http://www.fda.gov/downloads/drugs/guidancecomplianceregulatoryinformation/guidances/ucm4958 1.pdf
Risk Assessment: Mitigate Residual Risk at Each Stage
Conclusion
Product and process knowledge about all process validation life cycle stages must be captured, organized, managed, stored, and shared. It must also remain easily accessible to promote data-driven, science- and risk-based decision-making. Available, reliable data that can be evaluated statistically at any time ensures that each stage of the life cycle is in control. This enhances both regulatory compliance and product/process confidence, and improves product understanding.
Metrics developed may be submitted as defined in the FDA’s “Request for Quality Metrics” draft guidance. 16 Near-real-time trending of quality attributes, and process parameters ensures early signal detection and presents opportunities for manufacturers to act before a process failure occurs. The greatest benefits of a well-implemented life cycle KM system include reduction of product-remediation costs, lower cost of quality, and above all, consistent delivery of quality products to the patient.
- 16US Food and Drug Administration. Draft Guidance. “Request for Quality Metrics.” July 2015. https://www.regulations.gov/contentStreamer?documentId=FDA-2015-D-2537-0002&attachmentNumber=1&disposition=attachment&contentType=pdf