Best Practices for Deploying Real-World Evidence Solutions
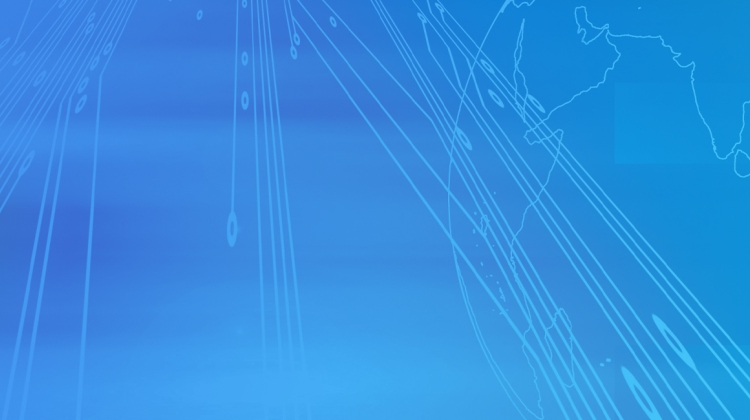
Real-world evidence (RWE) is clinical evidence regarding the usage and potential benefits or risks of a medical product derived from analysis of real-world data (RWD) relating to patient health status and the healthcare delivery.1 Real-world evidence helps healthcare companies better understand and establish stronger evidence of products’ performance, clinical value, and cost-effectiveness outside the controlled environment of clinical trials. Outcome-based studies are increasingly depending on real-world data and real-world evidence to speed up drug development and approvals, and ultimately reduce development costs.
- 1US Food and Drug Administration. “Use of Real-World Evidence to Support Regulatory Decision-Making for Medical Devices: Guidance for Industry and Food and Drug Administration Staff.” 31 August 2017. https://www.fda.gov/media/99447/download
Furthermore, when derived from real-world data such as medical data generated in hospitals, real-world evidence can provide additional insights into epidemiology, compliance, and costs, and therefore can help to satisfy the rising demand for information from payers, regulatory bodies, and healthcare providers regarding drug safety. In September 2020, former US FDA Commissioner Scott Gottlieb, MD, outlined real-world evidence’s impact on the clinical development, regulatory decision-making, and postmarket data collection of COVID-19 vaccines and treatments.2 He noted that real-world evidence provides flexibility for postmarket safety and effectiveness data collection, supports decision-making about patient care, is used to augment data sets already being accrued, and enables substantial improvements in the clinical care of COVID-19 patients in a relatively short period of time.
The real-world evidence market is expected to be worth $1.6 billion by 2024,3 and its value may possibly be greater than that due to effects from the COVID-19 pandemic. Real-world evidence solutions are available for drug development and approvals, market access and reimbursement/coverage decisions, clinical decision-making, medical device development and approvals, and other applications of relevance in the life sciences industry.
But how exactly is real-world evidence generated from real-world data? Are there specific quality aspects to be considered in the validation of real-world data and the tools utilized to generate real-world evidence used for regulated purposes? And how can GAMP® principles be used to validate the components and deliverables?
From Real-World Data to Real-World Evidence
Real-world data are routinely collected from a variety of sources4 ,5 ,6 including:
- Electronic health records (EHRs) and electronic medical records
- Claims and billing data
- Product and disease registries
- Patient-generated data, including in home-use settings
- Health-related apps and mobile devices
- Health surveys
- Observational studies
- Social media
Studies/analyses conducted on real-world data lead to real-world evidence. Such studies may complement the information collected and analyzed through a traditional clinical trial.7 For example, in 2018, blinatumomab was approved for the indication minimal residual disease (MRD)–positive acute lymphoblastic leukemia using data from a single-arm clinical trial that included a historical comparison group of retrospective data on patients collected from clinical sites.8
Current Challenges
If real-world evidence is used in a regulated context, the processes and tools used to generate the real-world evidence should be validated.
Operational challenges in real-world evidence generation include feasibility, governance, and sustainability issues. Among the key issues are the complexities of accessing and using multiple data sources that have different legal requirements for sharing data. Data anonymization is required to meet data privacy regulations, and efficient and timely delivery of data must be ensured.9
Technological challenges include differences in terminologies, data formats, quality, and content that exist across multiple databases, leading to heterogeneous data. Heterogeneity may cause significant problems when pooling multiple data sets from various populations to explore diseases, events, or outcomes.9
Process Overview
Because real-world evidence might be generated to answer a variety of questions, ranging from non-GxP-relevant market research to GxP-relevant clinical trial or pharmacovigilance support, the associated processes must have adequate controls in place for GxP-relevant real-world evidence generation while at the same time enabling flexible and efficient processing of all analysis requests. Examples of adequate control may include validation/qualification of platforms and computerized systems and independent double programming (multiple programmers using the same specifications and raw data to assess whether they achieve the same results).10
As the general process of generating real-world evidence cannot be exclusively associated with a single business process, it is essential to establish a robust product and process understanding for each project that generates real-world evidence. The risks associated with the usage of real-world evidence within the GxP-regulated business process are key to scaling life-cycle activities as part of the life-cycle approach and defining the required controls during the analysis. The general process of generating real-world evidence typically provides a framework and workflow to ensure only qualified/validated tools are used and project-specific risk assessments are performed.
The process to generate GxP-relevant real-world evidence from real-world data can generally be described in the following phases: analysis, build, and execution and reporting.
Analysis Phase
During the analysis phase, the following aspects must be documented and approved in, for example, a real-world evidence study/analysis protocol:
- Definition of the business question to be answered for intended use of the real-world evidence (e.g., for clinical trials, reimbursement, drug safety)
- Selection of the research approach (e.g., noninterventional study, analysis of social media), data source (e.g., EHR systems, product and disease registries) and methodology (e.g., population, exposure, and outcomes of interest)
- Approach to identify and minimize bias
During this phase, the required technology and the development and execution activities as well as potential challenges should be assessed at a high level. For example, a long-term study involving continuous monitoring of social media using artificial intelligence (AI) requires radically different approaches and controls than a one-time analysis of product registry data using traditional statistics. As stated previously, a risk assessment considering the supported business process should be performed and documented at this phase. Aspects such as audit trails of data changes or change control for continuously trained AI to ensure the results can be reproduced in cases of need should be considered in this phase.
Build Phase
During the build phase, the following aspects must be documented and approved in, for example, an real-world evidence study/analysis plan:
- Description of the sample size considerations for the study data source
- Formal definitions of exposure, outcomes, and other variables included in the analysis, including any manipulations/transformations that will be conducted
- Methods for dealing with bias, missing data, and other data issues
- Methods for analyzing and documenting the study outcomes
Real-world data analysis usually involves development of statistical programs and algorithms; therefore, all statistical programming deliverables should be developed according to processes established for statistical analysis in other GxP-regulated areas, such as clinical trial data analysis. Depending on the associated risks, practices such as peer reviews of code/algorithms and independent double programming may need to be developed and tested in the build phase.
Execution and Reporting Phase
After the successful build and testing of the real-world data analysis, the real-world evidence is generated. Depending on the intended use of the real-world evidence, the real-world evidence might be produced only once or repeatedly. The outcome and a summary of the build phase should be documented in a real-world evidence-study/analysis report. If the real-world data analysis is executed repeatedly, a maintenance plan might be required.
Responsibilities
The generation of real-world evidence requires a cross-functional team capable of critical thinking to identify and adequately address all risks to patient safety, product quality and data integrity. Table 1 identifies roles and responsibilities for members of this team.
Data Sources
Just like in a traditional clinical trial, data quality in a real-world evidence analysis is of critical importance. A risk-based approach considering the specific regulatory use of the evidence, the overall data integrity of the entire regulatory-relevant data set, and, ultimately, the safety of the patient should be used to determine the necessary level of real-world data quality. The FDA has provided the following example in their guidance to illustrate this point:1
A specific registry might be leveraged for post market surveillance, but not be adequate to support a premarket determination of reasonable assurance of safety and effectiveness or substantial equivalence.
Real-world data are typically collected and aggregated for specific, nonregulated purposes, so an understanding of the strengths and limitations of the real-world data, and how these qualities potentially impact the relevance and reliability of the data in the context of the intended use, is critical. It should be noted that real-world data could be biased—for example, data from premium healthcare providers may not be representative of the entire population. Additionally, the qualification and the intentions of the people recording the data (patient, physician, clinical investigator, etc.) may introduce bias and/or affect the overall quality of the data. Recently, a COVID-19 hydroxychloroquine study published in Lancet had to be retracted because the findings were based on EHR data from inconsistent sources, compromising the overall quality of the combined data set. 11 ,12
If real-world data are used to generate real-world evidence intended to support regulatory decision-making, the following aspects might be considered in the selection of real-world data sources:
- Appropriate scope for the intended use
- Data integrity (primarily accuracy and completeness)
- Ability to verify data against source documentation
- Definitional framework (i.e., data dictionary)
- Whether the data are representative and generalizable to the relevant population
Real-world data may be provided directly by organizations that collect and process them, or they may be obtained from specialized real-world data providers that curate, aggregate, and clean or transform data received from healthcare providers or other sources. The following areas should be covered when auditing real-world data providers.
- Coverage/quantity: For example, patient coverage, sample size, representativeness, completeness
- Granularity/depth: For example, types of patient-level data, such as diagnoses, procedures, laboratory tests, quality of life, observations, and outcomes
- Accessibility: Data access and usage limitations, raw data sharing, data privacy
- Quality: Richness of the data, origins of the data, data-entry quality standards
- Legal issues: For example, permission to use data for secondary purposes
- Timeliness: Data-refresh frequency, historical coverage
- Technical quality: For example, system validation/qualification, IT processes, data cleaning and transformation processes
Role | Responsibilities |
---|---|
Business representative | • Definition of requirements and risk assessment • Review of the real-world data deliverable • Usage and further processing of the RWE within the business process, including archiving |
Data science representative | • Controlled data transformation and storage • Adherence to the real-world data-generation process • Development and testing of algorithms • Documentation of the development process |
IT | • Provisioning of qualified computing environment |
Quality assurance | • Auditing of data providers, storage providers, and tool suppliers |
It should be noted that it may not be possible to verify all data integrity aspects for real-world data sources because these sources are often anonymized. For example, it may not be possible to identify the patient or the reporter of the data, or data may not be “original” anymore because data are copied and transformed to be suitable for real-world data analysis. All data transformations should be clearly documented, and adequate controls should be in place to ensure the ALCOA+ (attributable, legible, contemporaneous, original, accurate, complete, consistent, enduring, and available)13 ,14 aspects of data integrity are not violated in the process.
As data sources can only be assessed against known intended data usages, documentation of the real-world data and real-world data vendor evaluation is important to enable further future usage of the data for new purposes. This documentation must be controlled by robust data governance processes that assess and document the appropriateness of the real-world data for each intended use, and control the access to the data.
Data Processing Platforms
Organizations often establish complex IT platforms to store and analyze real-world data. These platforms must establish data availability, provide tools for the development of analysis algorithms, and have adequate processing power that can be flexibly allocated to an individual analysis.
Data governance processes should be in place to define data availability aspects and requirements for each source of real-world data, such as:
- Need for data transfers, including requirements for transfer frequency and mode (incremental or full)
- Need for audit-trail data changes
- Type of database model (relational, object, graph, flat files, etc.)
- Type of data (structured, unstructured, semistructured, etc.)
- License and access model
Furthermore, the analysis of real-world data often requires a large amount of processing power; therefore, the real-world data/real-world evidence platform must
provide functionality to flexibly assign processing power (e.g., as provided by graphics processing units [GPUs]). The processing power must be usable for a potentially large set of development tools ranging from statistics software such as R and SAS to programing environments used in AI development such as Python, to “self-service” analysis tools intended for nontechnical end users. Often, specific additional libraries must be acquired and integrated in the analysis. In addition, visualization tools may be required to provide the real-world evidence in a format that facilitates decision-making or further processing.
The underlying infrastructure and supporting vendors for these platforms must be qualified following the principles as laid out in the ISPE GAMP® Good Practice Guide: IT Infrastructure Control and Compliance.15
- 2“Real-World Evidence: State-of-the-Art, Current Challenges and Future Vision.” Pharmaceutical Executive 40, no. 12 (30 November 2020). https://www.pharmexec.com/view/real-world-evidence-state-of-the-art-current-challenges-and-future-vision
- 3Meticulous Market Research Pvt. Ltd. “Real-World Evidence (RWE) Solutions Market Worth $1.64 Billion by 2024, Growing at a CAGR of 15% from 2019—Market Opportunity Analysis and Industry Forecasts by Meticulous Research®.” Global Newswire. 23 June 2020. https://www.globenewswire.com/news-release/2020/06/23/2052249/0/en/Real-World-Evidence-RWE-Solutions-Market-Worth-1-64-Billion-by-2024-Growing-at-a-CAGR-of-15-from-2019-Market-Opportunity-Analysis-and-Industry-Forecasts-by-Meticulous-Research.htm
- 4US Food and Drug Administration. “Real-World Evidence.” 30 November 2020. https://www.fda.gov/science-research/science-and-research-special-topics/real-world-evidence
- 5Malone, D. C., M. Brown, J. T. Hurwitz, L. Peters, and J. S. Graff. “Real-World Evidence: Useful in the Real World of US Payer Decision Making? How? When? And What Studies?” Value Health 21, no. 3 (2018): 326–333. doi:10.1016/j.jval.2017.08.3013
- 6US Food and Drug Administration. “Patient-Focused Drug Development: Collecting Comprehensive and Representative Input. Guidance for Industry, Food and Drug Administration Staff, and Other Stakeholders.” June 2020. https://www.fda.gov/media/139088/download
- 7Khosla, S., R. White, J. Medina, et al. “Real World Evidence (RWE)—A Disruptive Innovation or the Quiet Evolution of Medical Evidence Generation?” F1000 Research 7 (2018):111. doi:10.12688%2Ff1000research.13585.2
- 8Zettler, M., and T. Phillips. “RWE in Regulatory Submissions for Cancer Drugs.” Cardinal Health Focus Magazine website. 2020. https://www.cardinalhealth.com/en/services/manufacturer/biopharmaceutical/real-world-evidence-and-insights/resources-for-real-world-evidence-and-insights/focus-magazine/rwe-in-regulatory-submissions-for-cancer-drugs.html
- 9 a b Cave, A., X. Kurz, and P. Arlett. “Real-World Data for Regulatory Decision Making: Challenges and Possible Solutions for Europe.” Clinical Pharmacology and Therapeutics 106, no. 1 (2019): 36–39. doi:10.1002/cpt.1426
- 10Randall, A., and B. Coar. “Risk-Based Validation in Clinical Trial Reporting: Focus on What Matters Most.” PharmaSUG 2018 Paper SI-02. Accessed 11 March 2021. https://www.lexjansen.com/pharmasug/2018/SI/PharmaSUG-2018-SI02.pdf
- 1
- 11Mehra, M. R., S. S. Desai, F. Ruschitzka, and A. N. Patel. “RETRACTED: Hydroxychloroquine or Chloroquine with or without a Macrolide for Treatment of COVID-19: A Multinational Registry Analysis.” Lancet S0140-6736(20)31180-6 (22 May 2020). doi:10.1016/S0140-6736(20)31180-6
- 12Toumi, M., M. Biernikiewicz, S. Liang, et al. “A Critical Analysis and Review of Lancet COVID-19 Hydroxychloroquine Study.” Journal of Market Access and Health Policy 8, no 1 (2020): 1809236. doi:10.1080/20016689.2020.1809236
- 13World Health Organization Expert Committee on Specifications for Pharmaceutical Preparations. “Fiftieth Report, Annex 5: Guidance on Good Data and Record Management Practices.” WHO Technical Report Series no. 996. 2016. https://Www.Who.Int/Medicines/Publications/Pharmprep/WHO_TRS_996_Annex05.Pdf
- 14International Society for Pharmaceutical Engineering. ISPE GAMP® Guide: Records and Data Integrity. North Bethesda, MD: International Society for Pharmaceutical Engineering, 2017.
- 15International Society for Pharmaceutical Engineering. GAMP® Good Practice Guide: IT Infrastructure Control and Compliance, 2nd ed. North Bethesda, MD: International Society for Pharmaceutical Engineering, 2017.
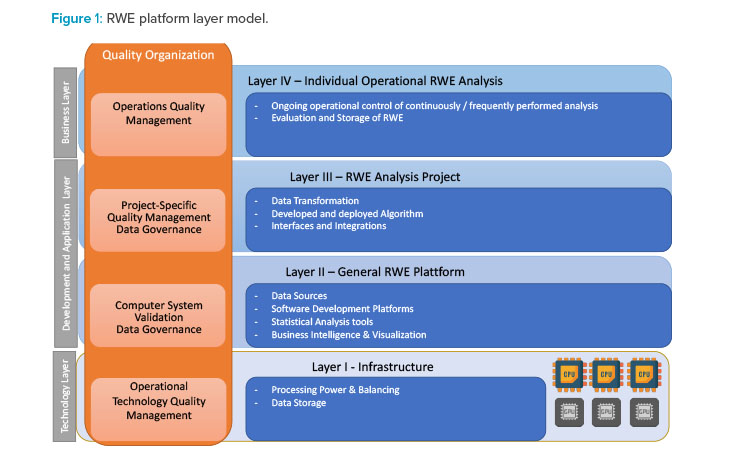
Quality Oversight
From a computerized system validation perspective, real-world evidence platforms are similar to platforms used in clinical trials, where a set of tools and systems sup-ports an individual clinical trial. Therefore, a similar approach could be used as described in the GAMP® Good Practice Guide: Validation and Compliance of Computerized GCP Systems and Data (Good eClinical Practice).16 Figure 1 presents a four-layer model for the Real-world evidence platform.
Layer I provides qualified infrastructure with a special focus on supplying the required processing power for individual real-world evidence activities as well as ade-quate data storage for real-world data and real-world evidence. Processing power might be provided by central processing units (CPUs) or GPUs. The qualification and process for provisioning GPUs are especially important, as they are often the only areas where GPUs might be used for GxP-relevant data processing.
Layer II establishes a tool set for the development of analysis algorithms using reliable data sources. The tool set also encompasses all tools required for data ingestion, as well as reporting and visualization tools required to provide the real-world evidence in the required format. This tool set should be validated/qualified to ensure these tools are fit for the development of the algorithms to analyze real-world data. The aspect of change control is of critical importance because most of these development tools are improved constantly or could be modified with additional functionality or libraries. As noted previously, reliable data sources are needed; furthermore, all performed qualification and evaluation activities should be recorded as part of the platform qualification/validation. Risk assessment of the data sources, the tool set, and the development process should always consider that real-world data analyses with direct and significant impact on patient safety and/or product quality could be developed and implemented.
Layer III uses the underlying layers to develop and deploy the algorithms, including all necessary data transformations for an individual analysis, following defined processes for software development and project management as applicable. The algorithms may be interfaced with other systems. Algorithms or solutions should be validated following the principles outlined in the GAMP® 5 Guide17 but also build upon the validation activities performed in Layer II. The primary focus of algorithm validation should be the correctness and reliability of the developed algorithm and the associated risks derived from the supported business process. The GxP risk of the business process should drive the extent of the controls that are required. For example, while an algorithm for a GxP critical area might require double programming and additional independent review, an algorithm for an area with low GxP risks might just be independently reviewed. Similar controls that have been implemented in other areas, such as statistical analysis of clinical trial data, can be adapted to real-world data analysis. The risk and the complexity of the analysis are also the key drivers for determining the required evidence and documentation that need to be established.
Layer VI includes real-world evidence use by business function, including adequate storage. For algorithms that are executed continuously or frequently, adequate operational controls must be established as for other computerized systems. These controls may address topics such as backup and restore, business continuity, training, and so on.
Throughout all layers, adequate control of data and tools (e.g., user access and user rights) must be established and maintained to ensure data integrity is maintained throughout the entire process.
Risk Assessment
Organizations often establish a central data science department that provides real-world data real-world data/real-world evidence services for the entire organization, including GxP- and non-GxP-relevant requests for real-world data analysis. As with any other software or computerized system, algorithm- and code-based real-world evidence systems require risk assessments to appropriately identify and design the required controls, and to scale and justify the validation efforts. Because real-world data analysis can be done in various ways using statistics and/or AI, and because the resulting real-world evidence can support all business processes regardless of their regulatory relevance or relation to product quality or patient safety, every RDE analysis project must receive a careful risk assessment. The vast majority of these projects should be classified as bespoke software (GMPS Cat. 5) because they include the development of custom code. A clear definition of the intended use of the real-world evidence and sufficient, documented user requirements, including the required data sources, form the basis for the risk assessment.
Risk assessments need to be performed for:
- All platforms and tools
- All data sources and providers
- All analysis projects and their support of business processes (intended use)
- Data transfers and data flows (including interfaces)
The risk assessment for platforms and tools should be performed as part of computerized system validation processes and activities. It should be noted that a significant number of tools are open source or are provided by vendors that are not familiar with GxP requirements. The tools used in real-world evidence generation are also used in a number of other industries that are not as regulated as our industry. GAMP 5 provides robust guidance for such risk assessments and can also be applied to open source software (see “Guide for Using Open Source Software [OSS] in Regulated Industries Based on GAMP” in Pharmaceutical Engineering, May/June 2010.18
As outlined earlier, the quality of the real-world data is of key importance. Risk assessments must determine the level of qualification required for the data providers and determine the reliability of the data itself. These risk assessments should be based on data integrity aspects, such as ALCOA+, and data privacy aspects; and issues with biased data must be included. Further guidance on data life cycles and data governance can be found in the GAMP® Guide: Records and Data Integrity.14
Often, extraordinary large amounts of data must be collected, transferred, and stored for the generation of real-world evidence. The security and integrity of the data during these activities must be ensured. Access control and possibly encryption in transit, as well as encryption at rest, may be required. A robust data governance framework is therefore advisable.
Obviously, not all real-world data analysis projects have that same risks, and each should be evaluated individually. In particular, analyses resulting in data for regulatory submissions and analyses related to patient safety or product quality must be reliable and trustworthy, and the generation of the real-world evidence should be traceable and/or repeatable.
Conclusion
As the use of real-world evidence for regulated purposes grows, the need to validate the tools and processes used to generate real-world evidence also increases. The validation approach outlined in this article, which adopts concepts from the validation of statistical analysis, AI, and clinical trials, and is based on GAMP® guidance in combination with a robust data governance framework, will facilitate regulatory compliance and, even more important, reliable and trustworthy real-world evidence.
- 16International Society for Pharmaceutical Engineering. ISPE GAMP® Good Practice Guide: Validation and Compliance of Computerized GCP Systems and Data (Good eClinical Practice). North Bethesda, MD: International Society for Pharmaceutical Engineering, 2017.
- 17International Society for Pharmaceutical Engineering. GAMP® 5 Guide: A Risk-Based Approach to Compliant GxP Computerized Systems. North Bethesda, MD: International Society for Pharmaceutical Engineering, 2008.
- 18Kaufmann, M., M. Ciolkowski, A. Hengstberger, et al. “Guide for Using Open Source Software [OSS] in Regulated Industries Based on GAMP®.” Pharmaceutical Engineering 30, no. 3 (May/June 2010). https://ispe.org/pharmaceutical-engineering/may-june-2010
- 14