Interpretation of Variance Components for Blend and Content Uniformity
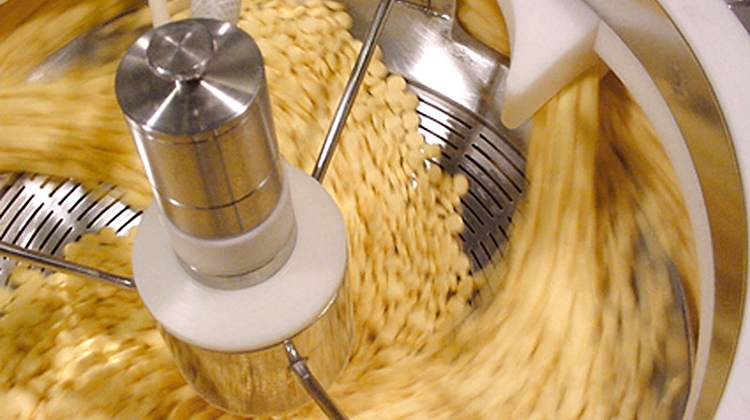
Despite introducing modern analytical technology to assess blend uniformity, many companies are still using traditional blend sampling thieves and wet chemistry to assess blend homogeneity. The use of statistically based sampling plans allows variance component analysis to be conducted on both blend and dosage unit data. This article shows how various combinations of blend and dosage unit variance components (“within-location” and “between-location”) can be interpreted to identify potential root causes for homogeneity issues, including sampling bias, and how they can be mitigated.
Sampling Plans
In August 2013, the US Food and Drug Administration (FDA) published a Q&A that included the expectation to test a set of three replicate samples that are taken from at least 10 locations in the blender when assessing blend uniformity.1 The FDA also expects that variance component analysis (VCA) be performed on the data to demonstrate uniformity of the mix throughout the blender.2 ,3 In the 2013 communication, the FDA stated that USP Chapter <905> “Uniformity of Dosage Units” should not be used to release commercial batches of solid oral dosage forms, recommending instead the use of statistically based acceptance criteria to ensure that USP Chapter <905> is passed throughout the product life cycle.
The use of statistically based sampling plans allows variance component analysis and comparisons to be performed on blend uniformity and content uniformity data of the subsequent dosage forms made from the blend. The standard deviations based on percentage of intent for blend and percentage of label claim for unit dosage are broken down into within-location variance and between-location
variance. Within-location variance assesses the variability of replicate samples within a single location. Between-location variance assesses the variability across either (a) the sampling locations throughout a blender for the blend or (b) the sampling times throughout the compression or encapsulation/filling process. This technique can be applied at any stage of the product life cycle.
Statistically based sampling plans should be used to assess both blend uniformity and dosage form content uniformity, especially during formulation and process development. Sampling plans should take a minimum of three samples from each sampling location in the blender or sampling time point during the compression run. This allows statistical analysis to be performed on the data, and the results can be used to characterize the batch quality and assist in identifying potential causes for blend and content uniformity issues. For blend sampling, triplicate blend samples should be taken and assayed from at least 10 locations throughout the blender. Fewer locations can be used for smaller batches, with justification. The number of locations to be sampled for the dosage units depends on the batch size.2 , 4 However, at least triplicate samples should be taken from each location, including at the beginning and end of the run.
Blend Sampling Technique
Poor blend uniformity is often attributed to sampling bias, which is often the result of poor technique to extract the sample from the blender, and sub-sequent mishandling prior to analysis. Ideally, it would be convenient to use a single blend sampling procedure for all products (thief design, sample weight, sampling locations, etc.). However, there is no guarantee that a single sampling technique can be used across products. Different blends have different characteristics, which may require developing a product-specific blend sampling procedure. A “good faith” effort to develop a sampling technique should be demonstrated prior to defaulting to the use of dosage units as a surrogate for blend uniformity.
Developing a blend sampling technique may consider the following aspects:
- Minimize operator-to-operator variability by ensuring that operators taking blend samples are properly trained.
- Define a sampling plan that provides coverage throughout the blender and includes known areas of slow material movement in the blender (i.e., dead spots).
- Ensure the sampling technique considers the type of thief (plug or chamber, material of construction, etc.), as the type can impact thief performance and results. Plug thieves pull stationary samples and eliminate potential segregation of the sample as it flows into the chamber.
- Determine whether to wipe the thief between samples. Residual material inside of the thief should always be removed between samples.
- Define the angle of insertion and cavity chamber position in the blend for chamber thieves. Triplicate samples from each location should be taken close to each other, but the thief
- should never be inserted into the same spot multiple times.
- Ensure the sample size is one (1x) to three times (3x) the dosage unit weight. If sampling bias is still evident at 3x, it may be necessary to use a different type of thief and/or identify the smallest sample quantity beyond 3x that reduces sampling bias and provides data similar to the drug product results. One example where this may occur is for low-weight tablets (~50–100 mg). Sample sizes smaller than 150 mg tend to be more prone to bias, and 3x or greater quantities may be required.
- Assay the entire sample; do not subdivide the sample. If
- possible, discharge the sample directly into labeled, pre-tared containers to minimize powder transfers during sample preparation.
- Be aware of the impact that static charge may have on the sampling process. Grounding the bin prior to sampling may be required to allow the charge to dissipate.
- Develop detailed blend sampling operating procedures in addition to the sampling plan to ensure consistency and repeatability.
Interpretation of Blend and Dosage Unit Variance Components
Once a rigorous statistically based sampling plan is implemented (either replicated sampling for a blend or stratified sampling for a drug product), variance component analysis can be performed. The variation of the blend potency or the content uniformity of the dosage units can be broken down into between-location variation and within-location variation. Throughout this article, the total variation is noted as total variance or total standard deviation (i.e., the square root of total variance), the between-location variation is noted as between-location variance or standard deviation, and the within location variation is noted as within-location variance or standard deviation.
For balanced sampling plans (i.e., plans with equal number of samples at each of the locations), method of moments can be used to estimate the variance components. Assuming there are B locations, and n samples tested at each of the B locations for content uniformity, xij, i = 1, 2, ..., B; j = 1, 2, ..., n. The mean and variance at each location can be calculated as x̄i and s\(_{i}^{2}\), i = 1, 2, ..., B; the variance of between-location means is s\(_{x̄}^{2}\).
The within-location variance (\(ô_{ε}^{2}\)) can be estimated as the mean, (\(Σ_{1}^{B} s_{i}^{2}\))/B, of variances at each location. The between location variance (\(ô_{B}^{2}\)) can be estimated as the variance (\(s_{x}^{2}\)) of location means minus the within-location variance divided by n, (\(ô_{ε}^{2}/n\)). In summary:
Within-location variance: \(ô_{ε}^{2}\) = (\(Σ_{1}^{B} s_{i}^{2}\))/B
Between-location variance: \(ô_{B}^{2}\) = s\(_{x̄}^{2}\) - \(ô_{ε}^{2}/n\)
Total variance = \(ô_{B}^{2}\) + \(ô_{ε}^{2}\)
As an example, Table 1 illustrates the detailed calculation of variance components using the method of moments. For more general calculations of variance components calculations, please see references 3 and 5 .
Sample Potency(%) | Each Location (n = 3) | ||||
---|---|---|---|---|---|
Sample Location | A | B | C | Mean (x̄i) | Variance(s\(_{i}^{2}\)) |
1 | 97.8 | 97.2 | 95.2 | 96.73 | 1.80 |
2 | 97.9 | 99.1 | 98.9 | 98.65 | 0.39 |
3 | 98.1 | 101.8 | 103.3 | 101.07 | 7.01 |
4 | 99.8 | 103.0 | 101.7 | 101.49 | 2.47 |
5 | 99.7 | 100.9 | 100.3 | 100.31 | 0.32 |
6 | 98.6 | 100.8 | 99.2 | 99.54 | 1.25 |
7 | 99.8 | 98.8 | 98.8 | 99.12 | 0.31 |
8 | 102.7 | 100.4 | 105.9 | 102.98 | 7.61 |
9 | 101.2 | 102.0 | 103.6 | 102.26 | 1.42 |
10 | 97.4 | 99.2 | 101.0 | 99.20 | 3.23 |
Variance of location means: s\(_{x̄}^{2}\) | 3.49 | ||||
Variance (within-location): \(ô_{ε}^{2}\) = (\(Σ_{1}^{B} s_{i}^{2}\))/B | 2.58 | ||||
Variance (between-location) = \(ô_{B}^{2}\) = s\(_{x̄}^{2}\) - \(ô_{ε}^{2}/n\) | 2.62 | ||||
Variance (total): \(ô_{T}^{2}\) = \(ô_{B}^{2}\) + \(ô_{ε}^{2}\) | 5.21 | ||||
Standard deviation (within-location): sqrt(\(ô_{ε}^{2}\)) | 1.61 | ||||
Standard deviation (between-location): sqrt(\(ô_{B}^{2}\)) | 1.62 | ||||
Standard deviation (total): sqrt(\(ô_{T}^{2}\)) | 2.28 |
It is useful to plot blend and drug product content uniformity data at the start of analysis, as plots can identify variability and trends in the data. Scatter plots for scenarios often encountered have been previously published.6 Figures 1–4 are examples of plots that can be associated with low within-location and between-location variances (i.e., a uniform blend), high within-location variance (poor mixing on a unit dose scale—poor micromixing), high between-location variance (hot and cold spots in the blend—poor macromixing), and both high within-location and between-location variance (incomplete mixing).
- 1US Food and Drug Administration. “Questions and Answers on Current Good Manufacturing Practices, Good Guidance Practices, Level 2 Guidance—Production and Process Controls.” CDER/OC Office of Manufacturing and Product Quality: CGMP Subject Matter Contacts, 6 August 2013. https://www.fda.gov/drugs/guidances-drugs/questions-and-answers-current-good-manufacturing-practices-production-and-process-controls
- 2 a b Garcia, T., J. S. Bergum, J. K. Prescott, and R. W. Tejwani. “Recommendations for the Assessment of Blend and Content Uniformity: Modifications to Withdrawn FDA Draft Stratified Sampling Guidance.” Journal of Pharmaceutical Innovation 10, no. 1 (March 2015): 76–83.
- 3Searle, S. R., G. Casella, and C. E. McCulloch. Variance Components. New York: Wiley, 1992.
- 4Bergum, J. S., T. A. Parks, J. K. Prescott, R. W. Tejwani, J. Clark, W. Brown, F. J. Muzzio, S. Patel, and C. Hoiberg. “Assessment of Blend and Content Uniformity. Technical Discussion of Sampling Plans and Application of ASTM E2709/E2810.” Journal of Pharmaceutical Innovation 10, no. 1 (March 2015): 84–97.
- 5Pinheiro, J. C., and D. M. Bates. Mixed-Effect Models in S and S-Plus. New York: Springer, 2000. p. 528.
- 6Prescott, J. K., and T. P. Garcia. “A Solid Dosage and Blend Content Uniformity Troubleshooting Diagram.” Pharmaceutical Technology 25, no. 3 (March 2001): 68–88.
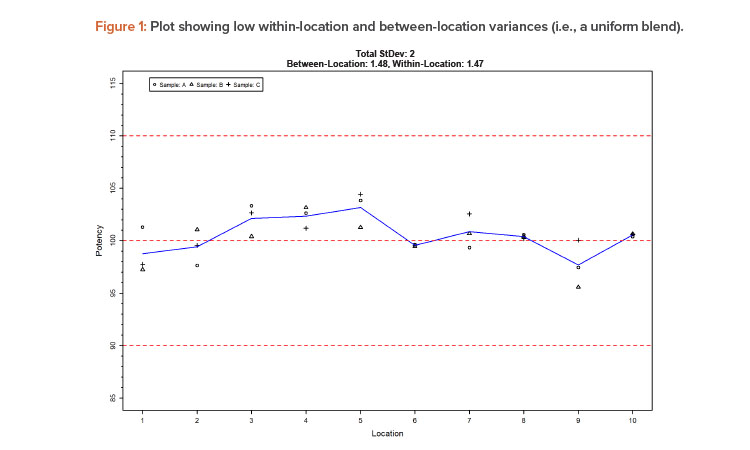
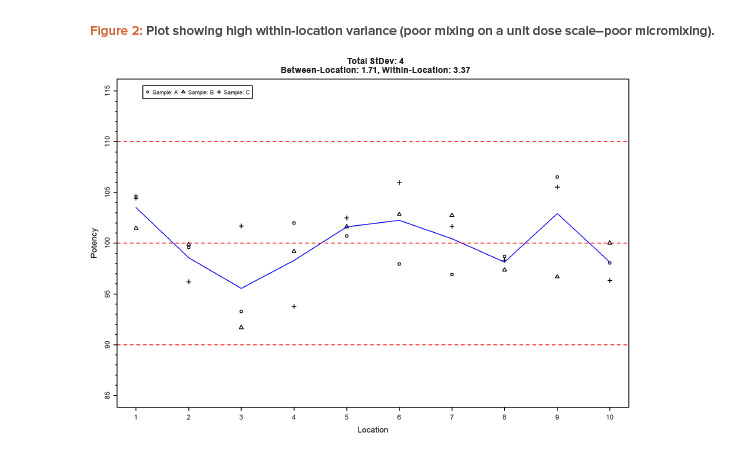
Note that the assignment of “high” or “low” labels for variance magnitude in this article is subjective. The reader should consider product risks for “high” and “low” labels for variance components, which may include the magnitude of the overall standard deviation, drug load in the formulation, historical behavior of the process, the drug’s therapeutic index, and other conditions. The dominant component is designated “high” and the minor component is “low.” The designation is qualitative and does not take into account the total variance. However, a “high” label for blend and content uniformity data with a high total standard deviation (for example 6%–7%) is more concerning than a “high” label for a batch with a total standard deviation of 3.5%. If the total variance can be attributed equally to within-location variance and between-location variance, both variance components are contributing to the overall variation for the product.
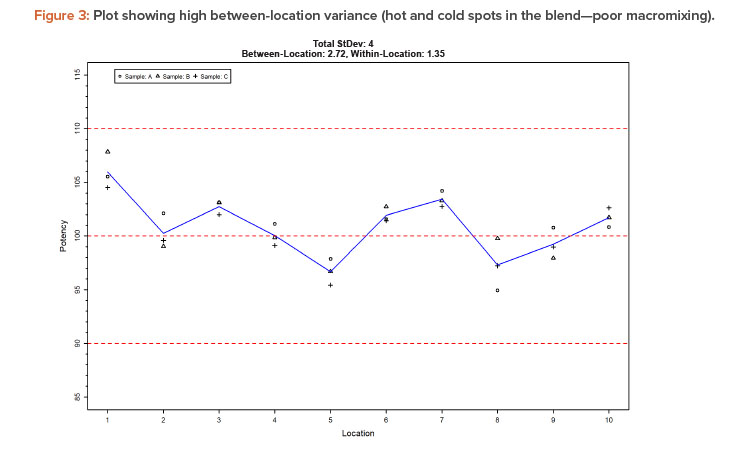
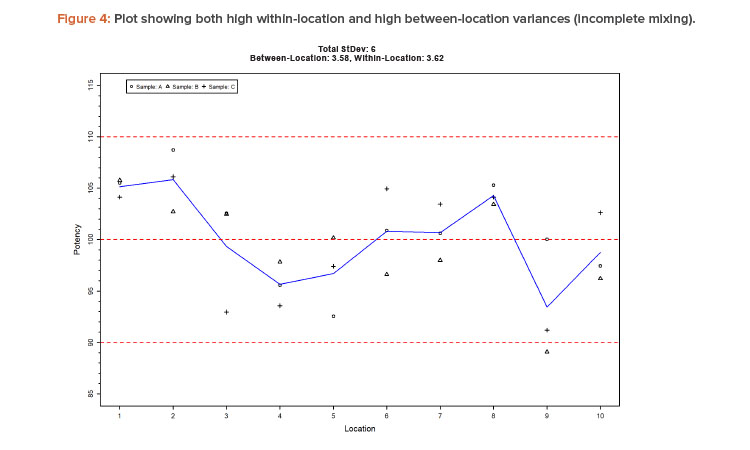
For the purpose of this article, standard deviation values are “rules of thumb”; standard deviations ≤3.0% for potency (based on percent label claim) are considered of acceptable uniformity for both blend and drug product uniformity without further analysis.2 In some cases, performing variance component analysis for batches with standard deviations ≤3.0% could be an overanalysis of the data. If the overall standard deviation is >3.0% for the blend and/or dosage units, variance component analysis can be useful to help identify potential causes contributing to the nonuniformity. It should also be noted that multiple lots of blend and dosage unit data should be included in the investigation.
Blend | Dosage Unit | Interpretation | ||
---|---|---|---|---|
Within Location | Between Location | Within Location | Between location | |
Low | Low | Low | Low | Both the blend and tablets are uniform. Variability between and within locations is low. |
Low | Low | Low | High | Blend is uniform. Segregation may occur during compression or filling at one or more locations. Plot stratified dosage unit data to identify where segregation is occurring. Implement controls and corrective actions. |
Low | Low | High | Low | Blend is uniform. Agglomeration may be missed during blend uniformity analysis. Further agglomeration of the drug substance during blend transfers to the filling/compression process due to the poor flow. Apply blend-mill-blend process; improve powder flow during transfer to reduce the agglomeration tendency. |
Low | Low | High | High | Blend is uniform, but the uniformity of dosage units may not be acceptable. Significant segregation and/or agglomeration of the drug substance may be occurring. Flow of the blend into compression/filling equipment may be poor. Plot stratified dosage unit data to identify signs of segregation or agglomeration. Implement controls and corrective actions. |
Low | High | Low | Low | Initial mixing is incomplete, with possible downstream mixing in the feed frame during the compression/filling process. There may be dead spot(s) in the blender, or a mechanically induced hot spot (e.g., drug substance fines settling in a fluid bed dryer or falling out of a filter) may create a drug enriched top layer in the blend. Identify the cause and implement controls and corrective actions for the mixing process. |
Low | High | Low | High | Mixing in the blender is incomplete, which carries over to the compression or filling operations. Neither the blend nor the dosage units have acceptable uniformity. Evaluate mixing efficiency. Plot blend and dosage unit data to identify where segregation may be occurring. Implement controls and corrective actions or optimize formulation. |
Low | High | High | Low | Blend is not uniform and likely has hot/cold spots. Some mixing may occur during the compression or filling process, but it is not enough to provide sufficient uniformity on a unit dose weight scale, resulting in high tablet-to-tablet variability for a given location. Flow of the blend into dies and filling equipment may be poor. Implement corrective actions controls for the blending step. |
Low | High | High | High | Blend uniformity is unacceptable due to incomplete mixing in the blender. The poor blend uniformity is carried over to the dosage units, producing tablets that do not have acceptable content uniformity. Plot stratified dosage unit data to identify the presence of agglomeration or whether segregation is occurring. Fixing the blend is the priority. Implement controls and corrective actions for the formulation and manufacturing process. |
High | Low | Low | High | Uniformity throughout the blender is acceptable. However, the high within-location variance may be due to blend sampling bias, agglomeration, or insufficient mixing on a unit dose scale. Segregation of the blend during material transfer (especially at the very beginning and/or end of the compression/filling of the batch) may be occurring. Plot stratified dosage unit data to identify whether segregation is occurring. Implement controls and corrective actions for the formulation and manufacturing process or the sampling procedure. |
High | Low | High | Low | Agglomeration of the drug substance occurs during mixing, which carries over to compression/filling process. Mixing on a unit dose scale is incomplete. Identify the cause and implement controls and corrective actions for the mixing process. |
High | Low | High | High | Uniformity throughout the blender is acceptable. Because the high within-location variance is carried over to the dosage units, blend sampling bias is ruled out; insufficient mixing on a unit dose scale may be an issue, and segregation of the blend during material transfer at very beginning and /or end of the filling or compression process may be present. Agglomeration of the drug substance may also be occurring. Plot blend and dosage unit data to identify where segregation may be occurring. Implement controls and corrective actions. Statistically based acceptance criteria should be used for batch release until process improvements and controls are implemented. |
High | High | Low | Low | Either the blend is not uniform and downstream processing (e.g., milling) results in uniformity, or the blend data are biased due to sampling technique/error. An investigation should be performed for the blending process and sampling method/procedures. Implement controls and corrective actions accordingly. |
High | High | Low | High | The uniformity of both the blend and dosage units is unacceptable. The formulation or process (or both) needs to be redesigned. |
High | High | High | Low | Blend is not uniform. Some mixing may be occurring in the feed frame, resulting in acceptable average potency across the batch. However, there is considerable tablet-to-tablet variability from a given location. Agglomeration and segregation may be occurring in the blend. The formulation, blending, and compression/filling steps must be improved. |
High | High | High | High | Blend and dosage units are not uniform. The formulation and/or blending and compression/filling processes are inadequate. The formulation, blending, and compression/filling steps need to be redeveloped. |
Table 2 presents combinations of blend and dosage unit within-location and between-location variance components and examples for the interpretation of various combinations of variance components. The table is not meant to be comprehensive, as other interpretations of the data may be applicable. As noted previously, the terms “high” and “low” are used in a relative manner in this article; they are only representative of a particular data set.
Sources of Variability and Their Mitigation
Potential sources of variability that can affect blend and content uniformity include incomplete mixing, segregation, raw material characteristics, ag-glomeration, and blend sampling bias.
Incomplete Mixing
Incomplete mixing results in a blend that has poor homogeneity. When this happens, the total standard deviation is high (for example, 5%–6% or greater) and may be split evenly between the within-location and between-location components. Table 3 identifies possible causes of incomplete mixing and strategies to resolve these issues.
Segregation
Segregation is a common issue that often occurs during material transfer, especially at the beginning (initial drop of blend onto the tablet press or filling equipment) or end of the batch during compression. Even though the initial blend is uniform, the expansion and movement of particles can result in separation of the drug and particles. This demixing often produces a high between-location variance component for the dosage units. Table 4 identifies potential causes of segregation and strategies to address these issues.
Raw Material Characteristics
Raw material attributes that have the potential to impact the mixing process and uniformity of the blends and drug product should be identified during formulation development. It is difficult to produce and maintain uniform blends without the proper characterization of the input raw materials.
It is important to select excipient grades that have similar physical properties (particle size, shape, and density) to those of the drug substance. Flowing powders with different particle size distributions can segregate. The particle size of excipients should be aligned with the drug substance, if possible.
Processes and equipment should also be designed to produce and maintain uniform blends. How the materials traverse through the process equipment train must also be understood. A drug-enriched layer may form on top of a blend due to the suspension of fine particles that settle out slower than granules at the completion of mixing. Fine particles of the drug substance that adhere to filter socks (e.g., fluid bed dryers and high shear mixers) can also form a drug-enriched layer on top of the mix. Subsequent blending (lubrication) may remix the fines. The final blend should be assessed for uniformity.
Cause | MitigationStrategy |
---|---|
Mixing process is insufficient to produce a uniform blend; possible demixing |
Optimize the blending process and process parameters |
Insufficient shear being applied to the powders with high cohesion |
Use a higher shear mixer. |
Limited room for the powder bed to expand during blending |
Adjust fill volume if operating at the high or low capacity of the blender. |
Poor formulation | Select excipient grades similar to the physical properties of drug substance, such as particle size. |
Cause | Mitigation Strategy |
---|---|
Equipment design and setup | Reduce vibration during compression and filling operations. Minimize free-fall distance between bulk container and tablet press, or other filling machine. Use decelerators to prevent free fall of powders during transfer. |
Manufacturing processes that directly blend the drug and excipients (e.g., direct compression); these processes are more prone to segregation, especially for low-dose products | Use wet and dry granulation processes to densify the blend and “lock” the drug substance and excipient particles together. Mill the granules to a consistent particle size distribution. |
Poor powder flow out of the bulk containers | Mill irregularly shaped particles. Use mass flow bins to eliminate rathole flowing. Vent powder transfer tubes. |
Agglomeration
Drug substances with sticky surfaces can agglomerate into larger particle sizes. The agglomeration can occur during the drug substance storage and in the process of drug product manufacturing. For the latter case, as the drug particles cascade and come into contact with one another, a snowballing effect produces agglomerated particles of the drug substance form. The inclusion of agglomerated particles in a single-dosage form could result in a superpotent dosage form.
Agglomerates can often be detected visually after the completion of blending operations. Passing the blend through a mill after the initial blend can reduce the impact that agglomerates have on content uniformity.
Blend and dosage forms with drug substance agglomerates may have high within-location variances, as only one of the replicate samples may contain them. Because superpotent tablets may not be commonly observed, and the impact of agglomeration can be diluted across the batch. The problem may go unnoticed, leading to the false conclusion that the batch quality is acceptable.
Potential sources of variability that can affect blend and content uniformity include incomplete mixing, segregation, raw material characteristics, agglomeration, and blend sampling bias.
Blend Sampling Bias
Sampling bias can occur when blend samples are removed from mixers. Causes of sampling bias range from differences in the physical characteristics of the formulation components to poor sampling techniques or poor methods to determine sample size. If the sampling technique is not properly developed, the blend samples are likely to be nonrepresentative of the true uniformity of the blend, resulting in a “bad blend, but good drug product con-tent uniformity” situation. When blend uniformity data are inconsistent among batches, even if there are no changes on material properties and process conditions, sampling bias may be the cause.
When blend sampling bias is present, the overall standard deviation is typically high (e.g., greater than approximately 4.5%), and most of the variation may be attributed to the within-location term. If the high blend within-location variance component is “real” (e.g., poor mixing on a unit dose scale), it will carry over to the dosage units, which will also have a high and dominant within-location variance component. As a result, blend sampling bias would be ruled out and the blend is not uniform. If the overall standard deviation for the dosage forms is low (e.g., less than approximately 3%), and that variation is distributed evenly for the between-location and within-location components, blend sampling bias is likely.
Assessing Blend and Content Uniformity Data
The primary purpose of blend uniformity analysis is to demonstrate that the drug is uniformly distributed throughout the blend, as reflected by the standard deviation of blend data. Although the mean for all samples is reported, the value is often off target due to sampling bias. For example, the preferential flow of excipients (and poorer flow of the drug substance) into the chamber of a sampling thief can result in a lower, albeit consistent mean. For this reason, the mean of dosage units tested (either in-process or during release testing) should be the only assay value of concern.
Blend and content uniformity should be assessed together. The assessment should include a thorough review of the data and information associated with the batch, as well as product history. Investigations for batches that are inconsistent with historical data should focus on events that may have occurred around the time of batch manufacture (e.g., a new lot of material, a change in the drug substance synthesis, different operators sampling the blend) that could incorporate variability into the process.
Data from each location should be plotted for both the blend and dosage units to assess the spread and any trends in the data.6 A side-by-side comparison of the means, standard deviations, and variance components for the blend and dosage units should be conducted to determine whether the results are consistent. In general, if the standard deviation is less than approximately 3%, the blend and dosage forms can be considered uniform. Standard deviations greater than 3% may be subjected to variance component analysis to identify potential areas for process improvement.
For the blend, a high between-location variance component implies that the blend is not uniform across all regions in the blender, signifying insufficient mixing on a macro scale. For tablets, a high between-location variance component implies that the dosage units are not uniform across the entire compression or filling process.
For both the blend and dosage units, high within-location variance components imply differences in the assay values for replicate samples within a single location. High within-location variance components can also be indicative of sampling bias.
Conclusion
Variance component analysis is a useful tool to identify root causes of blend and content uniformity issues, particularly during formulation and process development. Using statistically based sampling plans and proper sampling techniques ensures the proper data are collected to enable proper statistical analysis. Potential root causes can be investigated and mitigated, which could lead to process improvements.
Acknowledgments
In memory of our colleague and friend, Anthony Carella.