The Untapped Potential of AI & Automation in Pharmacovigilance
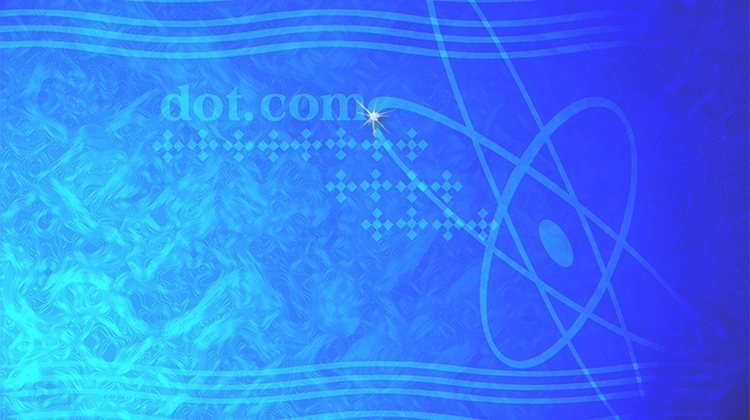
Between 2009 and 2019, the number of adverse events (AEs) for drugs and therapeutic biologic products recorded by the US FDA Adverse Event Reporting System (FAERS) increased more than 300%, from 490,032 to 2.19 million cases (as of 31 December 2019).1 Given the growth in case volume, the expanding number of sources for potential adverse events, and the complexity of new therapies, the pharmaceutical industry is exploring innovative technologies such as artificial intelligence (AI) to improve efficiency and quality in pharmacovigilance.
- 1US Food and Drug Administration. “FDA Adverse Events Reporting System (FAERS) Public Dashboard: Data as of December 31, 2019.” Accessed 13 March 2020. https://fis.fda.gov/sense/app/d10be6bb-494e-4cd2-82e4-0135608ddc13/sheet/7a47a261-d58b-4203-a8aa-6d3021737452/state/analysis
Safety surveillance needs to go beyond data-mining of spontaneous reporting systems and medical literature. Internal data sources can be supplemented with real-world data from electronic health records (EHRs), insurance claims, social media, and online communities. However, with traditional pharmacovigilance approaches, it is challenging—if not impossible—to incorporate, review, and analyze all these data in a timely manner.
AI can not only automate many manual, repetitive processes and enable greater consistency but also remove human bias and provide valuable insights for data scientists, medical reviewers, and physicians. They can understand their data in a much more comprehensive and extensive way than was previously possible.
By improving data quality, providing real-time data insights during processing, and enabling faster and more proactive signal detection, AI can have a huge impact on pharmacovigilance and patient protection. As drug safety shifts focus from operational tasks to more proactive risk management and greater data transparency among members of the pharma industry, patients, and providers, AI will become more essential. Applying AI in several key areas can help improve the end-to-end pharmacovigilance life cycle. See Figure 1 for an example of a unified solution.
Automating Case Intake
One of the most frequently discussed areas to apply AI is case intake. With the growing amount of information and numerous data sources containing mostly unstructured content, natural language processing (NLP) can be used to extract information quickly and efficiently. AI can be leveraged to convert content into structured data, autocode the data to dictionaries or code lists, and prioritize case findings based on seriousness, expectedness, and relatedness. Manual confirmations and adjustments to the case are fed back to the AI engine so that the machine learns and adjusts over time, thereby increasing accuracy and consistency.
Other areas where AI can improve case intake include literature monitoring, upfront review and data extraction from legal cases, and, potentially, using voice recognition to automate intake of adverse events from call centers.
Literature Monitoring
Many pharmaceutical companies struggle with retrieving and reviewing scientific and medical literature. Challenges include developing a good search strategy, understanding and extracting information from unstructured content, and converting content into adverse event data for automatic loading into a safety database.
AI can automatically, continuously, and extensively scan literature to find articles mentioning company products and determine whether the mentions involve an adverse event or multiple adverse events. Adverse event data can then be extracted from articles, imported into structured fields, and put directly into the safety database for review.
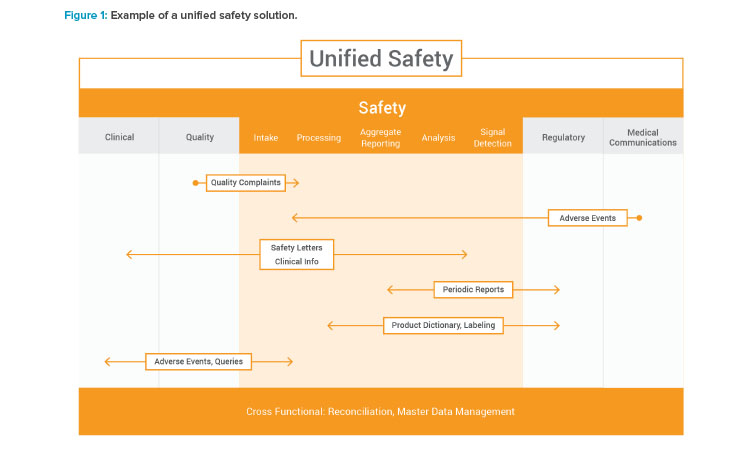
Legal Case Review
The volume of information received for legal cases can be considerable, and manual review of all source documentation can take days. Similar to medical literature review, AI can be used to efficiently “read and understand” legal case content to extract meaningful information.
Call Center Data Collection
Call centers are important collection points for adverse event information. Recent advancements in voice recognition technologies are enabling companies to consider using AI to extract adverse event information from call center recordings and load it into the safety database. Alternatively, AI can be used to quality-check data entered through manual data entry. The advances in technologies and the use of AI will reduce manual data entry, eliminate the need to email adverse event forms, and ultimately integrate call center systems with a safety database.
Streamlining Case Processing
Many aspects of case processing are still manual, requiring considerable overhead and creating compliance risk. Between 1 April 2014 and 31 March 2015, the UK Medicines and Healthcare Products Regulatory Agency (MHRA) conducted 47 inspections of pharma companies and found 27 critical, 169 major, and 155 minor findings. Of those findings, individual safety case report (ICSR) management was one of the top cited areas, especially for major and minor findings.2 Leveraging automation and AI can drive greater efficiencies, consistency, and quality in case management, narrative generation, and quality control, and improve future inspections.
Automating Narratives
Challenges with narrative writing include resourcing, consistency, timeliness with high-quality and potentially numerous data sources used as input, and high variability in the templates. In addition, for cases that have multiple follow-ups, the narrative can become disjointed and confusing. Automating narrative writing with natural language generation (NLG) provides greater quality and consistency within the narratives batch and reduces time to develop and finalize the narrative. AI can quickly and efficiently extract all relevant information, generate the narrative, and put it in the desired format or template. Built-in audit trails and version control also ensure that each version of the narrative is automatically tracked and stored for easy accessibility and comparison.
Quality Control
Manually comparing a source document with data entered in a database is a tedious and error-prone task. In addition, ensuring consistency across cases in the safety database is difficult. A “quality bot” can quickly and easily automate the quality review process by comparing the source data with the data in the safety database, looking for accuracy against the data entry guidelines and across the database for consistency with other similar cases—i.e., cases involving the same product, therapeutic area, or adverse event system organ class. Individual cases reviewed by the quality bot are assigned a quality score, and those that fall outside the threshold are flagged for review by a pharmacovigilance specialist.
AI is key to moving toward proactive and eventually predictive pharmacovigilance.
With access to better-quality data, medical professionals can better focus their efforts on medical review and deepen their understanding of the safety profile of their products.
Proactive Signal Detection and Risk Management
The goal of signal detection is to identify unknown causal associations between medicines and unexpected events. To achieve this, pharmacovigilance organizations must retrieve and periodically analyze safety data from various sources, including their global safety database, external data sources such as FAERS, VigiBase, and, potentially, real-world evidence sources.
AI can help rapidly analyze data across multiple data sources by many different factors, such as patient demographics, medical history, and medicines, in a fraction of the time comparable analysis using traditional methods would take. With AI, pharmacovigilance teams not only can have more comprehensive, real-time analyses but also gain more time to evaluate potential signals, make the appropriate signal validation decisions, and determine resulting actions to achieve better patient outcomes. After signals are detected and validated, AI can automate and manage the required risk management activities. A risk management plan can be created based on existing templates and related content from internal sources.
With the shift to proactive pharmacovigilance, expanding number of data sources, and technology improvements, it will be insufficient to report when an adverse event was identified. Regulators will also want to know, “When should you have known about the adverse event?” AI is key to moving toward proactive and eventually predictive pharmacovigilance.
Performing Analysis
In the digital era, more safety data are being collected, and AI is enabling companies to maximize the value of the information beyond interactive analytics. Using different data techniques and machine learning (ML), AI can quickly and efficiently analyze large and varied datasets. It can be used to solve complex problems or identify complex patterns, such as discovering the factors governing the association of a medicinal product and its effects on the population, linking certain compounds to a gene receptor, or flagging possible new indications for a product. Providing better epidemiological understanding of a disease can improve public health as well as patient safety and outcomes.
Analysis is only limited by the available data and one’s imagination. AI can analyze data much more extensively and quickly than traditional analytical methods. Stakeholders will have more time to review and validate the analysis and may be able to identify risks earlier.
Preparing Aggregate Report Content
Every product, regardless of development stage, has periodic reporting requirements. Pharmacovigilance teams need to submit to regulatory authorities development safety update reports (DSURs), periodic adverse drug experience reports (PADERs), periodic safety update reports (PSURs), or periodic benefit-risk evaluation reports (PBRERs). The exact reporting requirements vary by country, approval status, and stage of development for a medicinal product. The process of scheduling, planning, and preparing these aggregate reports is very time and resource intensive, but it can be made more efficient by AI applications.
Scheduling Line Listings and Tables
Periodic report content can be generated automatically by scheduling an automatic data pull from the safety database based on the product’s international birth date (IBD), the report data lock point, and other configurable variables. Once the information is retrieved, it can be placed in the correct template, ready for authoring.
Report Authoring
Using natural language generation, AI can draft sections of the report such as the worldwide approval status, changes to reference safety information in a periodic safety update report, signal overview for a periodic benefit-risk evaluation report, or actions taken for safety reasons since the last report. The most knowledge-intensive steps for aggregate reporting are analysis of the safety data and summarizing it in written form, which requires medical judgment. Eventually, as AI, natural language generation, and machine learning become more sophisticated, AI-written draft sections of the report will come to closely approximate the final version, requiring just review and minor adjustments by the human author.
Reconciliation and Master Data Management
Within a pharmaceutical or biotech company, adverse event information may originate from many areas, including clinical trials, product complaints, medical information call centers, and healthcare provider or patient reports. As the case is processed and more data are received, all the information sources must stay consistent and harmonious across the organization. When done manually, the data harmonization and cleaning process (i.e., reconciliation) is time consuming and a significant expense for most companies.
The implementation of a cross-development cloud platform with integrated AI processes could significantly reduce the amount of time spent on reconciliation. It could also improve the quality and consistency of the data, reducing risk for the organization.
The same technologies and automation methods can also be applied to data management. Having one source of common data that are used for multiple purposes has historically been difficult to achieve. Using AI to manage data changes and notifications could simplify data management and reduce overhead. For example, AI can help the regulatory team maintain one master company product dictionary that is also used by personnel in safety, clinical, and medical affairs. When the regulatory team changes or updates the master dictionary, other functional consumers automatically receive notifications and updates so there are no discrepancies. AI and automation can also help maintain label information, local licenses, and other types of data.
Unified AI Solutions
As cases are processed, there are many opportunities for AI to learn from decisions to improve processing of future cases. For example, a person contacts the call center to report they have a throbbing headache after taking a specific medication. The AI engine can process the information and suggest the symptom to be coded as a cluster headache. During medical review of several similar cases, the code is changed to a type of migraine headache. The AI engine can learn from the changes and in the future, for similar symptoms and cases, suggest coding it as a migraine headache.
With a unified pharmacovigilance solution, AI can continually improve, maximizing the value of the safety data and learnings.
Unfortunately, pharmacovigilance systems are typically fragmented, with many tools and technologies from a variety of vendors, and that can limit the AI engine from accessing data to learn and detect patterns. Cloud applications are designed to easily work together. With a unified pharmacovigilance solution supporting seamless, end-to-end processes, AI can continually improve, maximizing the value of the safety data and learnings.
AI Challenges and Risks
Though AI has many potential benefits, there are also concerns about the technology, including whether AI can correctly interpret data, how to explain AI-based decisions, and the amount and quality of data needed to train an AI engine.
Machine learning may require large, comprehensive data sets that are difficult to obtain or require significant human effort to create and maintain. In the pharmacovigilance industry, AI solutions can leverage public databases of adverse event data, such as FAERS, VigiBase, and EudraVigilance Data Analysis System (EVDAS). There are also emerging approaches that can help close data gaps, such as in-stream supervised learning where data are tagged in the course of an activity.3
To mitigate risks, companies can take a phased approach to adopting AI, such as initially using it to assist in decision-making before automating an entire process. For example, in case intake, AI can provide suggestions on coding and priority that would be reviewed and confirmed by a human. Once companies have confidence in the AI engine, they can enable automatic routing of cases for processing.
Conclusion
In recent years, there has been a lot of discussion about how to effectively use AI for pharmacovigilance. Many of these conversations have focused on solving the case intake problem, which is a significant burden and expense for safety organizations. However, the future of AI for pharmacovigilance is much broader as AI can be applied to many other areas.
AI has already made great strides in many other areas of life sciences, propelled forward by cloud technology. For example, it is advancing genomic diagnostics and helps radiologists detect breast cancer in medical images.4 ,5 For pharmacovigilance, cloud technologies, automation, and AI will enable companies to realize the greatest value from their safety data and drive better insights and transparency so that we—as patients and consumers—know that the medications we may need are safe and effective.
- 2FDA News. “Critical Findings in MHRA Inspections Increase for Second Straight Year.” Published 29 January 2016. https://www.fdanews.com/articles/175094-critical-findings-in-mhra-inspections-increase-for-second-straight-year
- 3Horvitz, E. “Machine Learning, Reasoning, and Intelligence in Daily Life: Directions and Challenges.” Accessed 13 March 2020. http://erichorvitz.com/AmbientAI_Keynote.pdf
- 4Dias, R., and A. Torkamani. “Artificial Intelligence in Clinical and Genomic Diagnostics.” Genome Medicine 11, no. 70 (2019). doi:10.1186/s13073-019-0689-8
- 5Stempniak, M. “AI Fails to Beat Radiologists in Large Study, But Pairing the Two Proves Prolific.” Radiology Business. Published 2 March 2020. https://www.radiologybusiness.com/topics/artificial-intelligence/ai-fails-beat-radiologists-mammography